Volastra:
Short Circuiting Cancer's Chaos
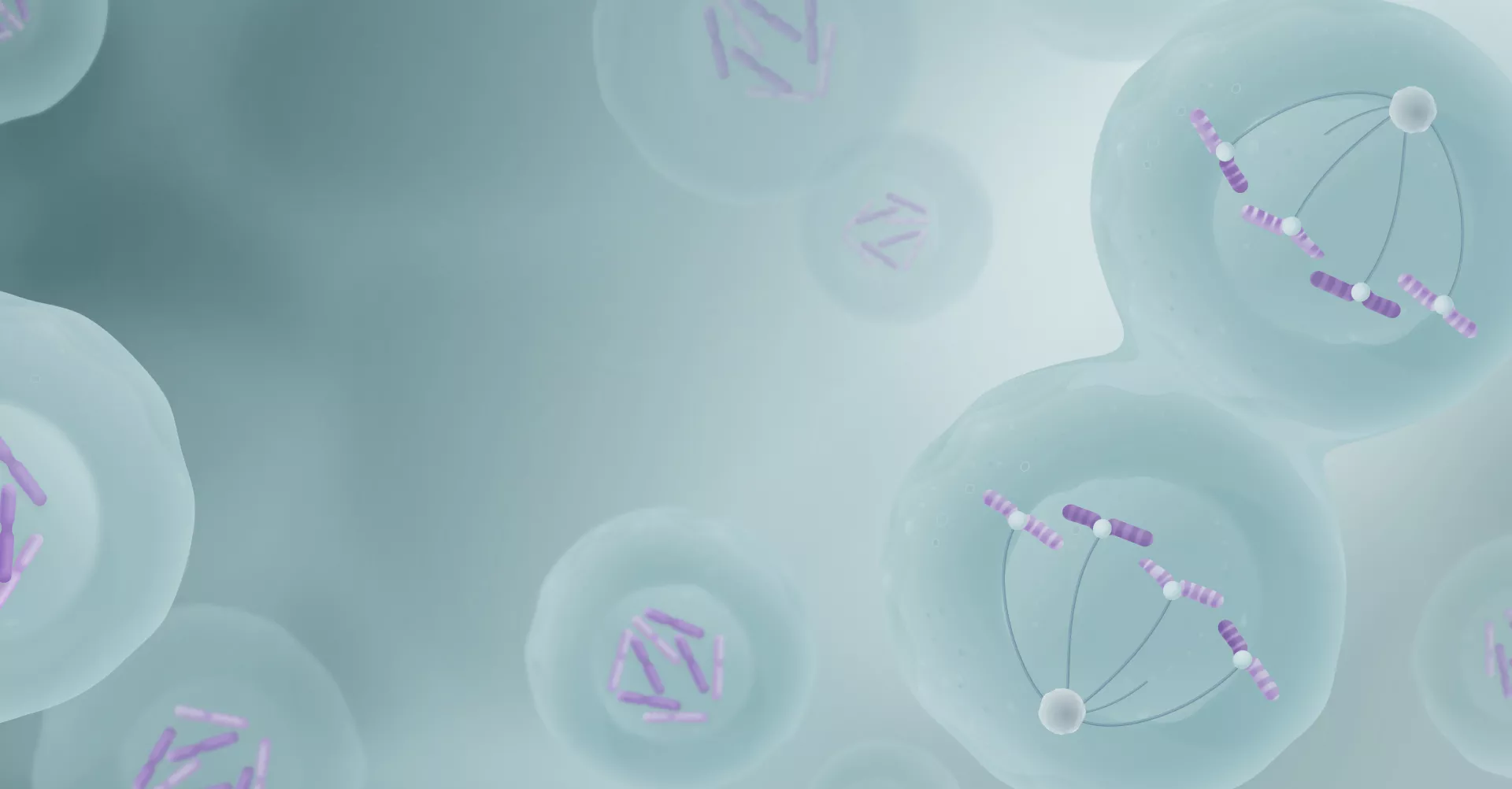
Volastra is an NYC-based clinical stage biotechnology company dedicated to the discovery and development of oncology therapeutics targeting chromosomal instability, or CIN, a key vulnerability of cancers.
WHAT IS CIN?
A hallmark of cancer, CIN refers to an increased rate of chromosome segregation errors during mitosis. The increased error rate drives structural and numerical changes in the genome which enhance heterogeneity. This heterogeneity gives cancer its ability to adapt and evade the body’s defenses and current medical therapies.Cancer’s unique vulnerabilities
For well over 100 years, researchers have considered the genomic chaos propagated by cancer cells’ abnormal chromosomes to be an inevitability of the cancer biology.
However, recent advances in science and technology have drastically changed our view of CIN. Simply put, cancer cells with chromosomal instability have key differences from normal human cells. We now understand that these differences are vulnerabilities unique to cancer cells that can be therapeutically targeted.
At Volastra, we are building on the seminal discoveries of our world-class founders and scientific advisors.
Our pioneering research has allowed us to understand CIN and design new medicines for patients with a wide range of difficult-to-treat cancers.
drug development
Using our innovative CINtech research strategy, combining proprietary technology, screening techniques, and computational modeling, we are able to measure and characterize chromosome instability in unique ways. This allows us to identify CIN-specific targets matched with new biomarkers to help us select the patients that may benefit most from our therapies.
Volastra’s CINtech lens
We are also applying CINtech to leverage large oncology data sets of well characterized cancer specimens that have been created over the past decades. By using our proprietary insight as a lens through which to interrogate this vast amount of information in ways never done before, we are able to make novel discoveries around cancer cell vulnerabilities.
Our research seeks to exploit the vulnerabilities of chromosomally unstable cancers through two complementary approaches.
Synthetic lethality and immune activation.
Our synthetic lethality approach seeks to directly kill cancer cells by identifying targets that are required by chromosomally unstable cells for survival but not by normal cells.
Our immune activation approach seeks to indirectly kill cancer cells, by identifying targets that re-awaken the immune sensing of chromosomal instability, a process that is actively thwarted in cancer.
VIEW OUR PIPELINEVolastra Therapeutics Announces Appointment of David P. Southwell as Chief Executive Officer
Volastra Therapeutics, a clinical-stage cancer biotechnology company, today announced the appointment of David P. Southwell as Chief Executive Officer.
Volastra Announces Initial Data from First-in-Human Phase I/II Trial of Novel KIF18A Inhibitor VLS-1488 to be Presented at 2025 ASCO Annual Meeting
Volastra Therapeutics, a clinical-stage cancer biotechnology company focused on chromosomal instability, today announced preliminary safety and efficacy data from its ongoing, first-in-human Phase I/II trial of its novel, oral KIF18A inhibitor, VLS-1488.
Volastra Therapeutics Announces the Appointment of Co-Founder Samuel Bakhoum, M.D., Ph.D., as Chief Scientific Officer
Volastra Therapeutics, a clinical-stage pharmaceutical company developing innovative cancer therapies targeting chromosomal instability, today announced the appointment of Volastra Co-Founder, Samuel Bakhoum, M.D., Ph.D., as its Chief Scientific Officer (CSO).